OpenAI’s next-generation language model “Orion” appears to have hit an unprecedented bottleneck.
According to The Information, internal OpenAI employees report that Orion’s performance improvements have fallen short of expectations, with quality improvements being “much smaller” compared to the upgrade from GPT-3 to GPT-4.
Furthermore, they indicate that Orion isn’t more reliable than its predecessor GPT-4 in handling certain tasks. While Orion shows stronger language capabilities, it may not surpass GPT-4 in programming abilities.
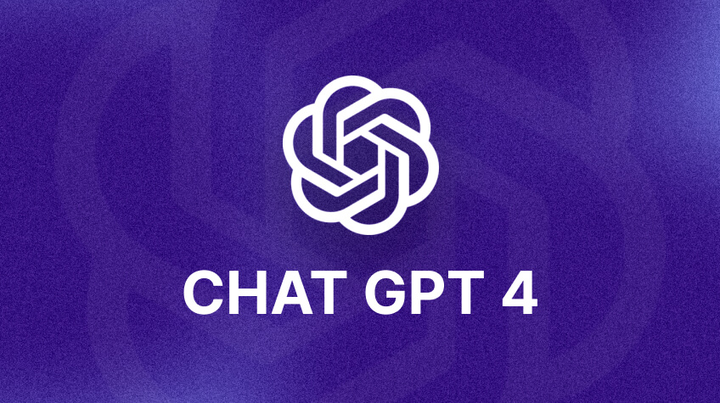
▲Source: WeeTech
The report points out that the supply of high-quality text and other training data is diminishing, making it increasingly difficult to find good training data and consequently slowing the development of large language models (LLMs) in certain aspects.
Moreover, future training will require more computational resources, financial investment, and electrical power. This means the costs and expenses of developing and running Orion and subsequent large language models will become increasingly expensive.
OpenAI researcher Noam Brown recently stated at the TED AI conference that more advanced models might be “economically unfeasible“:
Are we really going to spend hundreds of billions or trillions of dollars training models? At some point, the scaling laws will break down.
In response, OpenAI has established a foundational team led by Nick Ryder, who oversees pre-training, to research how to address the scarcity of training data and determine how long large model scaling laws will continue to hold.
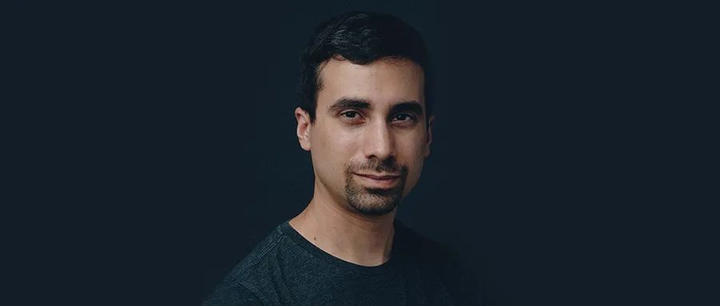
▲Noam Brown
Scaling laws are a core hypothesis in artificial intelligence: large language models can continue improving performance at the same rate as long as more data is available for learning and more computing power is available to facilitate training.
Simply put, scaling laws describe the relationship between inputs (data volume, computing power, model size) and outputs – how much performance improves when we invest more resources in large language models.
For example, training large language models is like producing cars in a factory. Initially, the workshop is small with just a few machines and workers. At this stage, each additional machine or worker significantly increases production because these new resources directly translate into increased production capacity.
As the factory grows, the production increase from each additional machine or worker begins to diminish. This might be due to more complex management or increased difficulty in worker coordination.
When the factory reaches a certain size, adding more machines and workers may have very limited impact on production. At this point, the factory may be approaching limits in land, power supply, and logistics – increased input no longer produces proportional output increases.
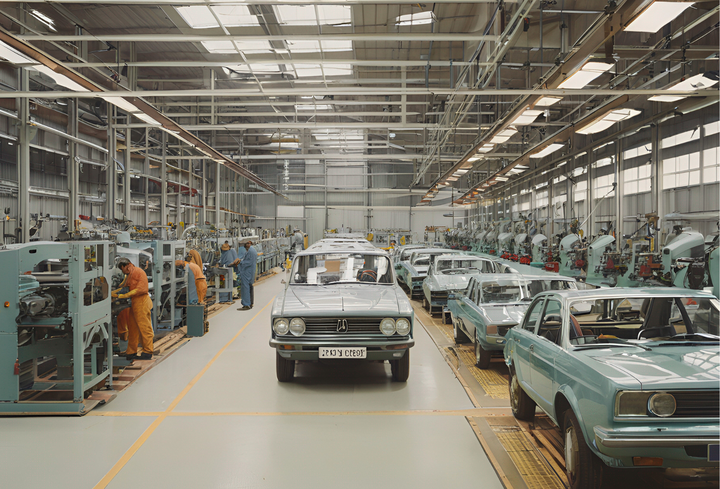
This is where Orion faces its challenge. As model scale increases (similar to adding machines and workers), performance improvements may be very noticeable in early and middle stages. However, in later stages, even continuing to increase model size or training data volume may yield diminishing returns – this is the so-called “hitting the wall.”
A recent paper published on arXiv also suggests that with growing demand for public human text data and limited existing data volume, large language models’ development is expected to exhaust available public human text data resources between 2026 and 2032.
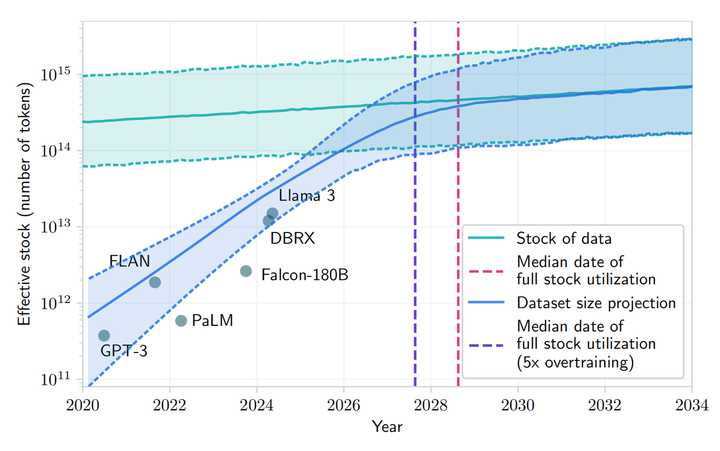
▲Source: arXiv
While Noam Brown pointed out the “economic issue” of future model training, he opposes the above viewpoints. He believes that “AI development won’t slow down anytime soon.”
OpenAI researchers largely agree with this perspective. They believe that while model scaling laws may slow down, AI’s overall development won’t be affected thanks to optimizations in inference time and post-training improvements.
Furthermore, Meta’s Mark Zuckerberg, OpenAI’s Sam Altman, and other AI company CEOs have publicly stated they haven’t reached the limits of traditional scaling laws and are still developing expensive data centers to improve pre-trained model performance.
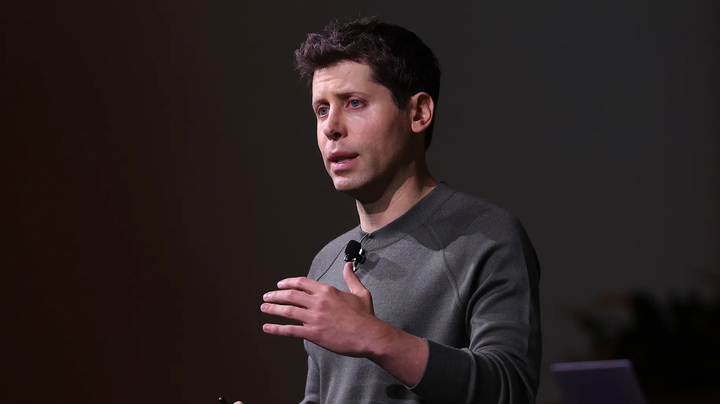
▲Sam Altman (Source: Vanity Fair)
OpenAI’s VP of Product Peter Welinder also stated on social media that “people underestimate the power of test-time computation.”
Test-time computation (TTC) is a machine learning concept referring to computations performed during inference or prediction on new input data after model deployment. This is separate from training phase computations where the model learns data patterns and makes predictions.
In traditional machine learning models, once trained and deployed, they typically don’t require additional computation to make predictions on new data instances. However, some more complex models, like certain types of deep learning models, may need additional computation at test time (inference time).
For example, OpenAI’s “o1” model uses this inference pattern. In fact, the entire AI industry is shifting focus toward a model of improving models after initial training.
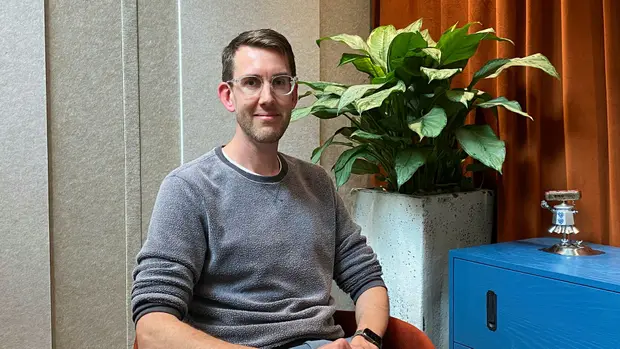
▲Peter Welinder (Source: Dagens industri)
Regarding this, OpenAI co-founder Ilya Sutskever recently acknowledged in a Reuters interview that improvements in the pre-training phase – using large amounts of unlabeled data to train AI models to understand language patterns and structures – have plateaued.
Ilya stated that “the 2010s were the era of scaling, and now we’re back in an era of exploration and discovery,” noting that “scaling at the right scale is more important than ever.”
Orion is expected to launch in 2025. OpenAI’s decision to name it “Orion” rather than “GPT-5” perhaps hints at a new revolution. Although temporarily constrained by theoretical limitations, we still anticipate this “newborn” with its new name to bring fresh breakthroughs to AI large models.